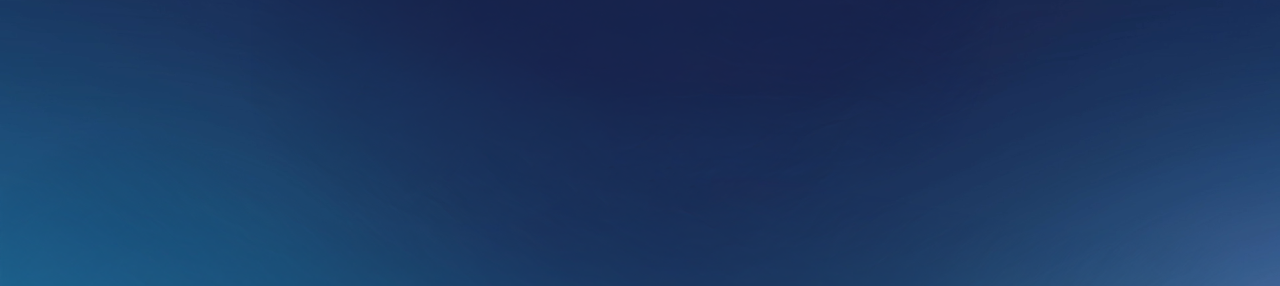
Persichetti Research
- Institute Scientist
- Assistant Professor, Departments of Rehabilitation Medicine and Radiology, SKMC
Memory & Perception Laboratory
50 Township Line Road
Elkins Park, PA 19027
The Memory and Perception (MAP) Lab studies how memory and perception systems support diverse cognitive functions. The lab focuses on two specific domains: 1) How people recognize different places (e.g., kitchens, beaches, gyms) and navigate within and between them; and 2) how conceptual information about things in the world (e.g., ‘dogs’, ‘chairs’, and ‘people’) and abstract ideas (e.g., ‘love’, ‘hope’ and ‘justice’) are represented in the human mind and brain. We use a combination of methods that includes functional MRI (fMRI), behavioral testing, and eye tracking. The lab also works to advance fMRI methods to provide researchers with tools that can better characterize individual differences in functional brain networks and noninvasively measure brain responses in humans that are closer to the single-neuron measurements achieved in animal models.
Research Projects
Recognizing Places & Navigating Through Them
People with topographical disorientation get lost and disoriented easily, even in familiar environments, and they can have trouble recognizing places (i.e., landmark agnosia). Many people who are post stroke or traumatic brain injury report symptoms of topographical disorientation. The MAP Lab seeks to advance therapeutic interventions aimed at alleviating symptoms of topographical disorientation by studying the neural systems that support place recognition and navigation in humans. We primarily focus on three regions in human cortex that respond preferentially to images of places and during tasks that require people to recognize places or navigate through them. A central assumption has been that all three of these regions directly support navigation. However, Dr. Persichetti and colleagues proposed instead that these cortical place (or “scene”) processing regions support three distinct computational goals (and one not for navigation at all): (i) The parahippocampal place area supports scene categorization, which involves recognizing the kind of place we are in; (ii) the occipital place area supports visually guided navigation, which involves finding our way through the immediately visible environment, avoiding boundaries and obstacles; and (iii) the retrosplenial complex supports map-based navigation, which involves finding our way from a specific place to some distant, out-of-sight place. A central goal of ours is to apply what we learn by precisely characterizing how the brain supports sophisticated cognitive processes, like recognizing places in the world and navigating around, to neurorehabilitation efforts.
Representation of Knowledge About the World
Storing knowledge about the world as concepts and organizing those concepts into categories is a core feature of human cognition. Categorization allows us to generalize knowledge across different experiences and to retain seemingly infinite facts about things in the world. A common framework for studying how categories are represented in the mind is to assume that they are comprised of concrete (e.g., “dog,” “hammer,” “carrot”) and abstract (e.g., “love,” “justice,” “intelligence”) concepts. In The MAP Lab, we use behavioral methods to understand how mental representations of knowledge are organized and we use fMRI methods to learn how the brain supports concept knowledge. For example, Dr. Persichetti and colleagues have been working to map the representational space of all concepts with an emphasis on the lesser studied domain of abstract concepts. In a recently published study, they showed that abstract concepts have a taxonomic structure with clear categorical boundaries. Unsing behavioral data collected from over a thousand participants while they made similarity judgements about a set of abstract words, the researchers then created a representational similarity matrix based on those judgments and used a clustering analysis to identify categories within the representational space. They then validated those categorical boundaries in a separate set of participants, using automatic semantic priming. These results demonstrate that abstract concepts, like concrete concepts, are taxonomically organized. Follow up work is being done to identify the dimensional space of all concepts and study the neural correlates of conceptual knowledge. As we continue this work at MRRI, we hope that it will inform rehabilitation approaches for people who are struggling with memory problems or aphasia due to dementia or stroke.
Dissociating Cortical Layer-specific Responses to Perception, Memory, & Action
Understanding how feedforward information from primary sensory cortices and feedback information from “higher order” brain areas are organized across cortical layers in regions that are involved in perception, memory, and learning processes is fundamental to understanding sophisticated cognitive functions and treating related mental disorders. We know that feedforward information from primary sensory and motor cortices, and feedback information from higher-order brain regions are dissociable across cortical layers. However, conventional fMRI methods that measure Blood oxygenated-level dependent (BOLD) signals cannot reliably resolve layer-specific activation because BOLD measures signals from large draining veins on the pial surface instead of microvasculature distributed more evenly across cortical depths. A new method called vascular space occupancy (VASO) can resolve activation across cortical depths by measuring changes in blood volume instead of changes in blood oxygenation. For example, measuring BOLD responses in primary motor cortex (M1) while a person repeatedly taps their fingers together results in a “blob” of activation that does not reflect the known laminar organization of input and output circuitry in M1. By contrast, with VASO, we can dissociate neural responses evoked by finger tapping in the superficial layers of M1 that receive cortical input and the deep layers of M1 that send output to the spinal cord to support movement. We have further shown that, as predicted, simply imagining finger tapping while remaining still evoked responses in superficial cortical layers only. As the young field of layer-fMRI progresses, the MAP Lab will continue to use cutting-edge methods to dissociate feedforward and feedback information across layers of human cortex both within and beyond primary sensory and motor cortices, while connecting these layer-specific responses to cognition and behavior.
Parcellating Gunctional Brain Networks using Resting-state fMRI Data
Parcellations of resting-state fMRI data are widely used to create topographical maps of functional networks in the human brain. These brain maps can be very useful for understanding the organization of the human brain, tracking changes to brain networks over time (e.g., pre and post rehabilitation), and comparing brain networks between groups (e.g., autism spectrum disorder and typically developing individuals). However, they usually require large sample sizes to make them, thus creating practical limitations for researchers that would like to carry out parcellations on data collected in their labs. This limitation must be overcome to be able to track changes to brain networks in an individual going through neurorehabilitation therapy or to compare brain networks between small clinical groups. Thus, Dr. Persichetti and colleagues recently developed a method, called FunMaps, to perform flexible and data-driven functional parcellations of the brain to derive network maps with relatively small datasets. They have used this method to functionally map the anterior portions of the temporal lobe and to derive a group-specific functional network map in individuals with autism spectrum disorder. In the MAP Lab, we are currently adapting the method to create functional maps that are unique to individual brains so we can track changes to brain networks in individuals going through neurorehabilitation protocols. The FunMaps method is publicly available on GitHub.